AI Readiness Assessment for Businesses
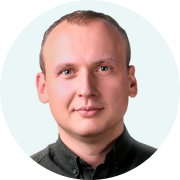
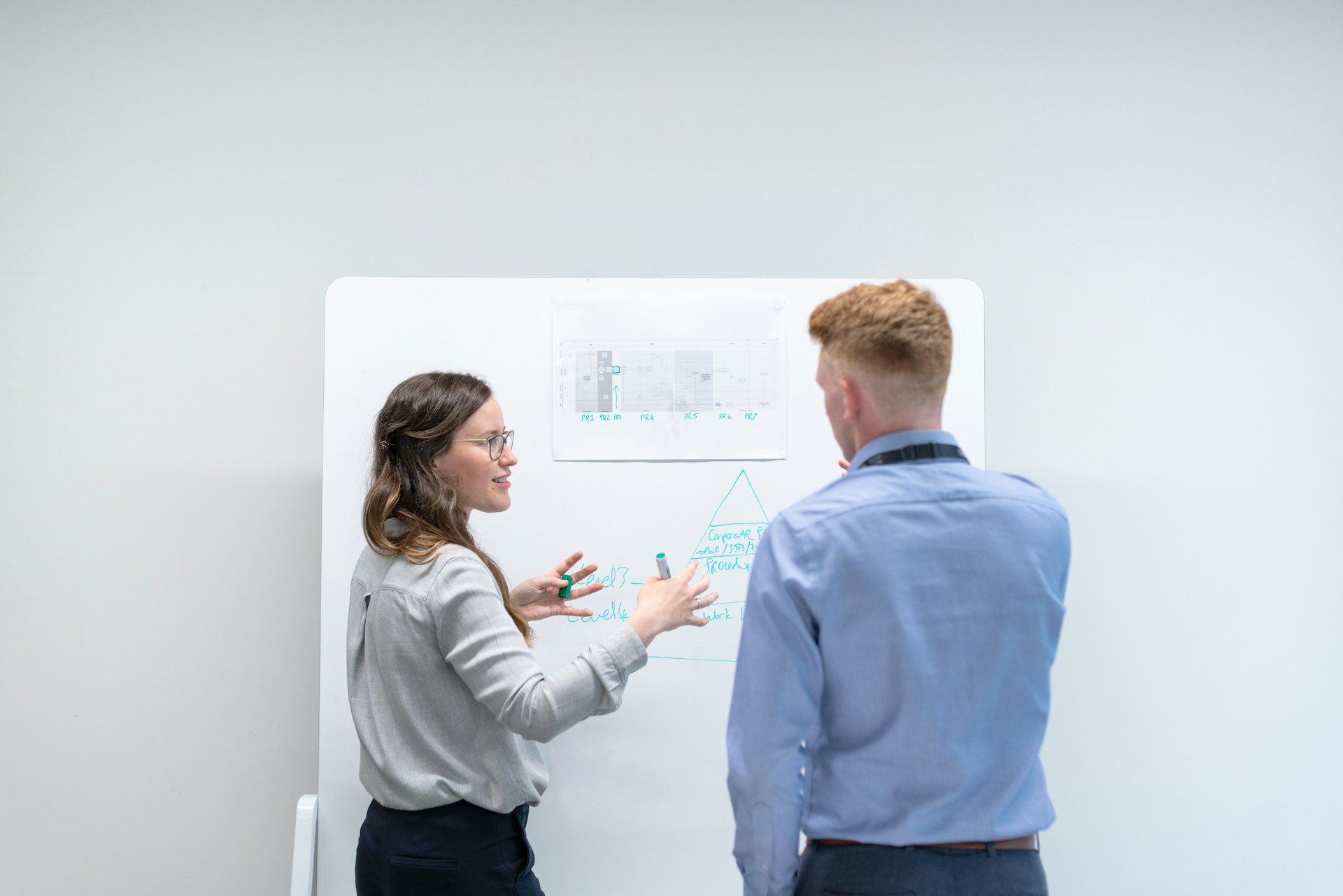
Artificial intelligence now touches everything from customer support chatbots to real-time demand forecasting. Yet many executives still ask the same question: Is our company truly ready to make AI stick? An AI readiness assessment answers it. By evaluating strategy, data, technology, and culture in one go, the assessment shows where an organization already excels and where gaps could stall progress.
Done well, the exercise goes deeper than a quick checklist. It distinguishes between broad readiness and the more specific AI assessment that gauges preparedness for large language models and related tools.
Only after that groundwork is clear does it make sense to look at numbers. In 2024, McKinsey reported that 78 percent of surveyed firms use some form of AI, but barely one-third have moved beyond isolated pilots. The disparity underscores why a systematic artificial intelligence readiness review is the shortest path from promising idea to working solution.
What is AI Readiness?
AI readiness is the composite state of a company’s strategy, technology, data, people, and governance that allows it to deploy artificial intelligence at scale. An organization is “ready” when it can move from proof-of-concept to production without crippling delays, hidden costs, or compliance surprises. A formal AI readiness assessment maps the current state of each domain, pinpoints gaps, and recommends concrete fixes.
Why AI Readiness Matters
Few projects test the entire business stack like artificial intelligence. Data pipelines, model hosting, privacy controls, and change-management plans must all click at once. Firms that complete an artificial intelligence assessment before investing in models see shorter development cycles and clearer return-on-investment targets because they budget and plan with real constraints in mind.
AI Readiness Assessment vs. Generative AI Assessment
A full AI readiness assessment reviews every class of machine-learning application: predictive analytics, computer vision, optimization engines, and more. A generative AI assessment narrows the lens to large language models, image generators, or code-generation tools. It tests for needs unique to generative systems, like GPU availability, prompt-engineering skills, guardrail policies, and intellectual-property risk, while still referencing the broader findings from the main assessment. Think of it as a focused chapter in the larger book of artificial intelligence readiness.
Common Misconceptions
- “We have data, so we’re ready.” Volume alone does not equal quality. Without clear lineage, labeling standards, and governance, even terabytes of data stay unusable.
- “An AI assessment tool will give all the answers.” Most AI assessment tools accelerate data gathering and scoring, but interpretation still depends on domain context and strategic goals.
- “Readiness is a one-time certification.” Markets shift, regulations tighten, and new models appear monthly. Continuous monitoring keeps the readiness score current.
- “Only tech giants need this.” Mid-market firms often benefit the most; a modest project that aligns with clear goals can out-perform a sprawling, under-funded initiative at a larger rival.
Why Your Business Needs an AI Readiness Assessment
Even the most compelling AI vision stalls when basic prerequisites are unclear. An assessment brings every stakeholder onto the same page about current capabilities, gaps, and realistic next steps. With that shared baseline, teams can commit resources with confidence and track progress against measurable targets.
Turning Ambition Into an Executable Roadmap
Plenty of companies announce AI pilots without first asking how each model supports revenue growth, cost control, or customer experience. An AI readiness assessment forces that alignment. It translates high-level goals into concrete milestones, such as data clean-up tasks, infrastructure upgrades, and talent plans, so leaders can track progress in quarterly terms, not vague aspirations.
Avoiding Expensive False Starts
Jumping into development before confirming artificial intelligence readiness often ends in rework: data that fails privacy audits, models that overburden legacy servers, or dashboards no one trusts. By revealing these gaps early, the assessment prevents sunk costs and preserves momentum. A modest investment in evaluation typically saves multiples of that amount in avoided redevelopment.
Allocating Budgets Where They Matter
AI budgets can disappear fast on GPU clusters, third-party APIs, or outside consultants. Readiness scoring highlights the highest-impact line items, allowing finance teams to channel funds toward the data pipelines, security controls, or skill programs that unlock the most value.
Managing Risk and Compliance Up Front
Regulations on data use, model transparency, and algorithmic bias are tightening worldwide. A structured AI assessment tests current controls against relevant standards, so compliance work runs in parallel with development rather than in crisis mode after launch.
Accelerating Time to Value
When strategy, technology, and governance are synchronized from day one, projects move from proof-of-concept to production faster. Teams spend less time fixing foundational issues and more time refining models. The result is earlier returns on investment and the confidence to expand into advanced workloads.
Core Components of AI Readiness
An AI readiness assessment weighs every layer of the organization that will influence success. The following components form a cohesive framework. Strength in one area seldom offsets weakness in another, so review each domain with equal rigor before committing resources.
Business Strategy and Objectives
Every readiness review starts with strategy. The question is not “Can we run a model?” but “Which outcomes deserve a model?” Map each potential use case, like fraud detection, demand forecasting, product recommendations, to a measurable business metric. This exercise keeps later technical debates anchored to revenue growth, cost reduction, or risk mitigation instead of abstract innovation goals.
Technological Infrastructure
Solid infrastructure converts strategic intent into operational reality. Assess on-prem and cloud capacity, network latency, and the ease of provisioning GPUs or other accelerators. For a generative AI assessment, verify that your stack supports large-language-model inference speeds, vector databases, and guardrail services. Gaps here often surface early through an AI assessment tool that benchmarks current performance against model requirements.
Data Infrastructure
Models learn only as well as the data they receive. Review data sources, lineage, quality rules, and access controls. Confirm that sensitive records are masked or tokenized and that retention policies meet regulatory demands. A robust artificial intelligence assessment also checks whether data is labeled in ways that align with target use cases. Unlabeled text or images delay time-to-value more than any hardware shortfall.
Operational and Process Efficiency
AI thrives in repeatable workflows. Examine how data moves from collection through preprocessing, training, deployment, and monitoring. Identify manual checkpoints that could create bottlenecks once models scale. Automation tools — CI/CD pipelines for ML, experiment-tracking platforms, feature stores — often deliver outsized benefits at this stage.
Financial Preparedness and ROI Consideration
AI projects fail as often from optimistic budgeting as from weak science. Build a cost model that covers hardware, software licences, talent, and ongoing MLOps. Then run ROI scenarios that factor in realistic adoption curves and model retraining cycles. Investors and boards respond better to clear payback periods than to high-level promises of disruption.
Securing C-Suite Buy-in
Convincing senior executives to fund AI isn’t just a budget discussion. It’s a credibility test: can the project team show that the initiative advances core objectives and manages risk at the same time?
Speak Their Language — KPIs, Not Algorithms
Executives care about revenue lift, cost avoidance, and risk mitigation. Frame insights from the AI readiness assessment in those terms. Instead of describing model architectures, translate findings into projected impact on gross margin, customer churn, or fraud losses.
Quantify the Gap and the Payoff
Use data from your AI assessment tools to show current capability scores next to target benchmarks. Then link each gap to an estimated cost or opportunity. When leaders see a clear delta — say, a 15-percent operating-cost reduction if data quality improves — they are more likely to back the remediation plan.
Present a Phased Roadmap
Most boards hesitate at open-ended AI budgets. Propose a phased rollout tied to specific milestones: data audit complete by Q3, pilot model in production by Q1 next year, full deployment across Region A by Q3. Clear checkpoints make funding requests tangible and reduce perceived risk.
Highlight Governance and Compliance Early
Executives know that AI failures draw regulatory and media scrutiny. Demonstrate that the readiness plan includes bias testing, privacy controls, and incident-response procedures. When leadership sees that compliance is baked in they gain confidence to proceed.
Offer Visible Early Wins
Select at least one use case that delivers measurable results in under six months — often a narrow automation task or a well-scoped forecasting model. Early wins validate the artificial intelligence readiness process, build momentum, and create internal advocates who can champion larger investments.
Step-by-Step AI Readiness Assessment
Rushing through the checklist exposes hidden costs later; turning it into a year-long audit drains momentum. The sequence below has proved practical for mid-market and enterprise clients alike and works with most assessment tools or in-house scorecards.
Step 1. Prioritizing AI Opportunities
Run cross-functional workshops to surface pain points that prediction, optimisation, or automation can solve. Score each idea on potential value versus implementation effort, then keep only the high-value, moderate-effort items.
Deliverables:
- Shortlist of 3–7 high-impact use cases, each mapped to a business KPI
- (optional) Two-axis value/effort matrix that visually ranks the candidates
Step 2. Detailed Technological & Data Infrastructure
Benchmark current compute (CPU, GPU, TPU), networking, orchestration, and CI/CD pipelines against the needs of each shortlisted use case. For a generative AI assessment, add vector-database readiness, content-filter guardrails, and prompt-engineering support. Audit data lineage, quality metrics, security controls, and retention rules; estimate the lift required to close each gap.
Deliverables:
- Infrastructure scorecard (current vs. required) with cost estimates to close gaps
- Data-quality and governance report detailing lineage, compliance status, and remediation tasks
- Architecture diagram showing how target models would slot into existing systems
Step 3. AI Feasibility Analysis
Prototype lightweight models using historical data to test predictive lift or content-generation quality. Assess integration complexity, change-management demands, and third-party risks. Parallel risk analysis evaluates privacy exposure, model drift likelihood, and supply-chain vulnerabilities in open-source libraries.
Deliverables:
- Feasibility report for each use case (technical, operational, regulatory)
Step 4. Mapping Effort & Feasibility Against ROI
Convert qualitative findings into a three-level scale (low, medium, high) for both effort and expected return. Plot every candidate on the matrix and draw a threshold line to separate Wave 1 (high-return, manageable effort) from later waves. Update the visual whenever costs or market conditions change.
Deliverables:
- ROI-effort matrix (executive-ready slide) identifying Wave 1 initiatives
Budget outline for Wave 1 with payback-period projections - Decision log documenting why some ideas were deferred or dropped
Step 5. Skill & Talent Gap Analysis
Inventory existing data engineers, ML engineers, domain experts, and product leads, then match roles to Wave 1 needs — prompt engineering, MLOps, model governance, synthetic-data expertise, and so on. Decide where upskilling is feasible and where outside hiring or a partner is faster.
Deliverables:
- Skills-to-requirements matrix highlighting gaps and critical roles
- Upskilling plan with timelines, budgets, and suggested training paths
- Recruitment/partnership brief outlining external talent requirements and onboarding steps
AI Readiness Checklist
A good checklist condenses the entire AI readiness assessment into a single view you can revisit before every funding round or project kickoff. Use the sections below as a living document; update scores and notes as your artificial-intelligence initiatives evolve.
1. Business Strategy
- Confirm each proposed model maps to a clear KPI.
- Verify executive sponsorship with a named budget owner and decision timeline.
- Document how success will be measured after deployment.
2. Technological Infrastructure
- Record available compute and network capacity, noting any provisioning lead times.
- List container orchestration, CI/CD, and monitoring tools already in place for ML workloads.
3. Data Infrastructure
- Catalogue data sources, quality scores, lineage tracking, and access controls for each target use case.
- Flag gaps in labeling, anonymization, or retention that could delay training or breach compliance rules.
- Assign owners and deadlines for data-clean-up tasks.
4. Operations & Process Efficiency
- Map the workflow from data ingestion through deployment and monitoring, highlighting manual checkpoints.
- Note any missing automation layers — feature stores, experiment tracking, or model registry integration.
- Outline an incident-response path for model failures or data-drift alarms.
5. Culture, Skills, and Change Management
- Compare current staff skills against project requirements: MLOps, prompt engineering, model governance, domain expertise.
- Identify upskilling opportunities versus roles that require external hiring.
- Draft a communications plan to prepare end-users for AI-driven process changes.
6. Financial Preparedness & Governance
- Build a cost model covering hardware, licences, cloud spend, external talent, and ongoing MLOps.
- Tie every budget line to an expected ROI window and track actuals post-launch.
- Capture compliance checkpoints and confirm audit responsibilities.
Keep this checklist visible and revisit it at each project milestone. Consistent, incremental updates turn a one-off artificial intelligence assessment into a living readiness score that guides portfolio decisions.
AI Assessment Tools
Selecting the right AI assessment tool depends on what you need to measure — enterprise-wide maturity, model-level performance, or compliance controls. Below are three practical categories, each with examples you can explore.
1. Cisco AI Readiness Assessment
Cisco AI readiness assessment delivers a web-based questionnaire that scores six pillars — strategy, infrastructure, data, governance, talent, and culture. The output is a dashboard highlighting gaps and suggested actions, useful for board presentations.
2. Scale AI Evaluation
Scale AI Evaluation focuses on benchmarking advanced language and vision models. It runs each model through standardized tasks, flags weak spots, and suggests data to close them — ideal for teams moving prototypes into production.
3. Galileo Evaluation Intelligence Platform
Galileo Evaluation Intelligence Platform monitors generative AI applications after deployment, tracking cost, latency, and accuracy while surfacing failure modes for quick fixes.
4. Arthur Bench
Arthur Bench is an open-source framework that lets teams A/B test large language models side by side, rating them on relevance, safety, and consistency. Useful when you must choose between GPT-4, Claude, or open-source alternatives.
5. AIF360
IBM AI Fairness 360 provides Python and R libraries with more than 70 bias-detection metrics and mitigation algorithms, helping teams document fairness as part of their AI readiness review.
6. Microsoft Responsible AI Toolbox
Microsoft Responsible AI Toolbox bundles error analysis, fairness checks, causal inference, and interpretability dashboards in one interface; it connects directly to Azure ML but runs locally as well.
Navigating AI Implementation Successfully
The real test of an AI readiness assessment comes after the slide deck: translating findings into working systems that deliver measurable gains. Moving from preparation to production involves disciplined project management, continuous measurement, and an appetite for iteration.
Actionable Roadmap
Start by pairing each high-priority use case with the gaps documented earlier. If data quality scored low, schedule enrichment work before model training; if network bandwidth limits GPU clusters, commit budgets for upgrades in the same quarter the pilot is slated to begin. Keeping this dependency chain visible prevents teams from coding models that infrastructure can’t yet support — one of the most common stumbling blocks in artificial intelligence readiness programs.
Pilot, Then Widen the Blast Radius
A focused pilot validates both the technical design and the change-management plan under real-world traffic. For example, a customer-service chatbot powered by a small language model can gauge call-deflection rates before the firm invests in a larger generative model. Collect performance metrics, user feedback, and operating costs; feed the data back into your AI assessment tool to update readiness scores. When the pilot clears target thresholds, roll the same governance templates and MLOps playbooks into the next business unit.
Monitoring, Feedback, and Continuous Improvement
AI systems drift; regulations evolve; usage patterns shift. Embed monitoring hooks into every deployment. Treat these metrics like any other key performance indicator: review them in weekly ops calls, assign owners, and budget time for remediation. A light-weight quarterly generative AI assessment keeps your compliance and security posture up to date without repeating the entire readiness study.
Avoiding Common Pitfalls
- Over-customizing too early. Enterprises often build bespoke pipelines before proving value. Use managed services or open-source starters first; customize only when the business case is clear.
- Ignoring change management. Even the most accurate model fails if users don’t trust it. Involve frontline employees in pilot testing, publish decision-logic explanations, and incorporate their feedback into model updates.
- Underestimating operational budget. Cloud inference fees, feature-store licences, and retraining cycles can erode projected ROI. Track these costs from the first sprint, adjusting business-case models as real numbers replace estimates.
- Treating compliance as an afterthought. Privacy reviews and bias testing added late in the cycle lead to code rewrites. Integrate governance checkpoints at every stage, from data ingestion to model deployment.
Final Words on AI Readiness Assessment
An AI readiness assessment replaces guesswork with evidence. By auditing strategy, technology, data, skills, and governance in one pass, it reveals exactly what stands between your current state and successful deployment — be that predictive models, recommender systems, or the newest wave of generative AI tools. Armed with clear deliverables and a phased roadmap, leadership can fund projects in confidence, teams can build on firm ground, and the business can capture value faster than rivals still experimenting in isolation.
Frequently Asked Questions
What is AI readiness, and why is it crucial for my business?
AI readiness is the combined maturity of your strategy, infrastructure, data practices, talent, and governance. Without it, prototypes often stall in the pilot phase, budgets overrun, or compliance risks emerge late. A structured readiness review ensures resources go to projects that can reach production and pay back the investment.
How does a generative AI assessment differ from a traditional AI readiness assessment?
A full readiness assessment covers every class of machine-learning workload — forecasting, optimisation, vision, and more. A generative AI assessment zooms in on large language models and content generators, testing for GPU capacity, vector search, prompt-safety controls, and IP safeguards. Think of it as a specialised chapter within the broader evaluation.
How can AI enhance my business operations?
Well-targeted AI raises revenue through personalisation, reduces costs via intelligent automation, and lowers risk by spotting anomalies early. Results vary by sector, but companies that align AI use cases with measurable KPIs typically see returns within the first year of production deployment.
What key factors must be evaluated before implementing AI?
Start with business fit — each model should tie to a clear KPI. Next, audit infrastructure for scalable compute; inspect data quality, lineage, and access controls; gauge skills and culture; and verify compliance with privacy and fairness rules. Neglecting any one pillar often delays delivery.
Should businesses build internal AI teams or partner with specialised firms?
The decision comes down to timeline, budget, and talent availability. Building in-house grants long-term control but requires sustained hiring and upskilling. Partnering with an experienced provider accelerates delivery and fills niche skill gaps immediately, while leaving knowledge transfer plans in place for the future.