AI in the Manufacturing Industry
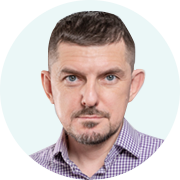
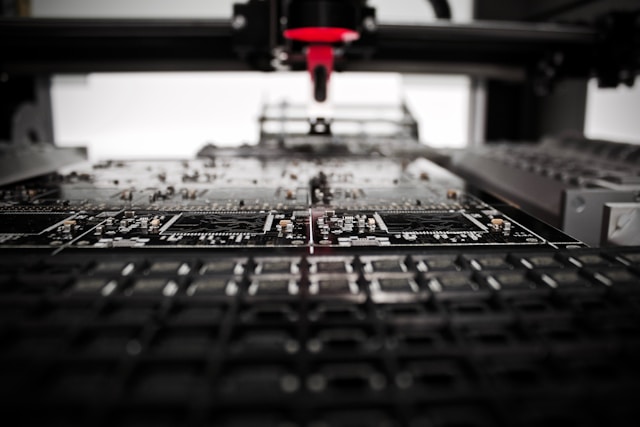
Every manufacturing leader knows the sting of an unexpected assembly line shutdown or a batch of products scrapped due to undetected defects. Traditionally, human inspectors catch only about 80% of production defects – meaning 1 in 5 flaws slips by. Forecasting demand has been equally challenging, with manual methods often missing the mark and leading to bloated inventories or stockouts. Today, these pain points are being tackled head-on by artificial intelligence solutions for manufacturing. AI-powered vision systems are now catching up to 99% of defects in real time. This isn’t hype or sci-fi – it’s the new reality of AI in the manufacturing industry.
We’ll explore three high-impact AI applications in manufacturing: AI-driven quality control & inspection, supply chain optimization & demand forecasting, and process optimization & automation. For each, we’ll look at the traditional challenges, how AI/ML is being applied, the tangible benefits, and key considerations for implementation.
AI-Driven Quality Control & Inspection
The Traditional Challenge
Quality control is the backbone of manufacturing, ensuring every product leaving the factory meets standards. Historically, this has relied on human inspectors and basic sensors to spot defects. However, manual inspection is slow, labor-intensive, and prone to human error. Even well-trained inspectors can become fatigued or miss subtle flaws. This means a significant fraction of faulty items escape detection, leading to rework, scrap, or worse – customer complaints and recalls.
Traditional automated systems can be faster, but they struggle with variation; a slight change in lighting or a new product variant might throw off their accuracy. The cost of poor quality in manufacturing can range from 5% to 30% of gross sales, which is a huge hit to the bottom line. Clearly, conventional methods of quality inspection have been a limiting factor, especially as product complexity and customization increase.
How AI is Applied
Enter AI-driven quality control, where computer vision and machine learning take center stage. Instead of relying on tired eyes or rigid rules, manufacturers are deploying AI-powered visual inspection systems that learn to recognize defects. Using high-resolution cameras and sensors, these systems capture images of products on the line and analyze them using deep learning models. For example, a neural network can be trained on thousands of images of “good” versus “bad” parts, learning to distinguish normal variations from true defects. Techniques like supervised learning are common – engineers feed the model labeled examples of defects so it can detect those patterns on its own. AI can also employ anomaly detection: the system learns the pattern of a perfect product and flags anything that deviates from that pattern, even if the specific defect was never seen before. This is incredibly useful for catching novel issues that weren’t explicitly anticipated.
What makes AI/ML in manufacturing quality control so powerful is its adaptive, high-speed analysis. AI vision systems don’t get tired – they can examine products 24/7, and often at blistering speeds. In fact, modern AI inspection cameras can analyze thousands of parts per minute, far beyond what a team of humans could handle.
Benefits and Examples
The practical benefits of AI-driven quality control are dramatic. First and foremost is accuracy – catching nearly all defects before products ship out. When an AI vision system is tuned well, manufacturers see scrap and defect escape rates plummet. For example, one implementation in an electronics factory boosted defect detection by 35% after introducing ML-based inspection. Such improvements directly reduce waste and rework costs. High accuracy also means improved product reliability and customer satisfaction.
Speed is another benefit. AI can inspect items much faster than humans, meaning 100% inspection of output becomes feasible. Traditionally, manufacturers might only sample-check products because checking every item was too slow; AI flips that equation, enabling real-time, 100% inspection without slowing production. This real-time defect detection allows for immediate corrections – if a trend of failures is spotted, the line can be stopped or adjusted at once. That kind of rapid feedback loop can prevent minor issues from snowballing into major production lots of faulty goods.
Consistency and objectivity are also key benefits. Unlike humans, AI doesn’t have good days and bad days – it applies the same criteria every time, eliminating variability. It can detect subtle flaws like hairline fractures or tiny paint blemishes consistently across every shift. AI doesn’t get distracted or tired, so the last unit on a 10-hour shift gets as thorough an examination as the first. This level of consistency is crucial for industries like automotive or aerospace where safety and precision are paramount.
Implementation Considerations
While the benefits are clear, implementing AI quality control isn’t as simple as plugging in some cameras. There are practical considerations and hurdles.
To train a robust computer vision model, you need lots of sample images of products, including examples of various defect types. Gathering and labeling this data can be time-consuming. Manufacturers often have to run pilot tests to capture enough defect images. Techniques like data augmentation or even simulation can help bolster the training dataset. Additionally, the AI needs to be tuned to avoid false positives – flagging every tiny anomaly isn’t useful if those anomalies don’t actually affect performance. It takes careful calibration to ensure the AI isn’t overly sensitive or too lax.
Another consideration is integration with existing processes. AI inspection systems must be integrated into production lines, often interfacing with conveyor systems and PLCs. If a defect is detected, the system might need to trigger a rejection mechanism or alert a human quality engineer. Ensuring this integration is smooth is key to reaping the benefits. There’s also the challenge of change management – line workers and inspectors must be trained to work alongside these AI systems. Rather than replacing humans outright, many companies re-focus their quality staff on managing the AI system, verifying its outputs, and handling the complex cases the AI flags.
Lastly, manufacturers have to consider the cost and ROI. AI inspection gear can be expensive upfront. However, for many, the ROI is justified by the reduction in scrap, rework, and warranty claims, as well as labor savings. It’s wise to start with a pilot on a critical process or product line – measure the improvements – and then scale up. Many firms start with AI solutions in manufacturing on one high-defect area to prove the value. They also need to ensure reliability: production environments can be harsh, so the AI hardware and models must be resilient and robustly tested.
Supply Chain Optimization & Demand Forecasting
Traditional Pain Points
Supply chain management has historically been a mix of art and science. Planners would use spreadsheets and basic ERP forecasts, combined with gut feel and experience, to predict demand and plan production. This often led to unpredictability and inefficiency. If forecasts were off, manufacturers either ended up with excess inventory sitting idle or faced stockouts of hot-selling items. Both situations are costly: excess stock ties up capital and may lead to waste, while stockouts mean missed sales and unhappy customers. A well-known phenomenon, the bullwhip effect, is when small fluctuations in demand get amplified upstream – due to delays and siloed information, a minor retail spike can cause wild swings in factory production orders. Traditional supply chains, with data locked in silos, made it hard to have one clear view of demand and supply. Each department might optimize locally but not globally. The result: inefficiencies like data silos, poor visibility, reactive decision-making, and slow response to market changes.
How AI/ML Improves Forecasting and Logistics
AI for supply chain comes in several flavors, but a core focus is demand forecasting – using machine learning to predict what customers will need, when and where, more accurately than ever before. Unlike simplistic methods that maybe do a straight average or a linear trend, AI demand forecasting can crunch vast amounts of data from diverse sources. Modern AI/ML models can analyze historical sales, seasonality, current orders, market trends, economic indicators, weather patterns, social media sentiment – any data that might be predictive – to find complex patterns in demand. These models learn non-linear relationships. By learning from the past and constantly adjusting, AI forecasts tend to get more accurate over time as more data comes in.
Another big advantage is real-time data processing. AI systems can continuously ingest data and update forecasts or trigger alerts. For instance, if a sudden trend is detected – say a sudden drop in demand in one region due to an unforeseen event – the AI can catch that and adjust production plans quickly, rather than waiting for a monthly review meeting. On the logistics side, AI helps with supply chain optimization by tackling problems like route planning, inventory optimization, and production scheduling. AI algorithms can optimize delivery routes for trucks, dynamically allocate inventory to different warehouses, and even reschedule factory production in response to changes. In essence, AI acts as the brain of a more responsive, data-driven supply chain, breaking down those silos by connecting data and decisions across the board.
Real-World Benefits
The impact of AI in supply chain and forecasting is quantifiable and significant. Companies that have embraced AI/ML for demand planning often report higher forecast accuracy, lower inventory costs, and faster response times. According to a McKinsey analysis, organizations implementing AI-driven demand forecasting have reduced forecast errors by 30% to 50%, which is huge. Better forecasts translate directly into leaner inventories – McKinsey noted inventory reductions of 20% to 50% in some cases, as AI helps pinpoint exactly what is needed and when. This means less money tied up in stock and less risk of products going unsold. Moreover, improved accuracy cuts down on the dreaded stockouts: lost sales due to out-of-stock situations can drop dramatically. These improvements strengthen the bottom line and customer service simultaneously.
AI also shines in making the entire supply chain more efficient end-to-end. For instance, better demand forecasts allow procurement to buy raw materials just in time, avoiding overstock of components. Production schedules can be optimized to smooth out peaks and troughs, improving factory utilization. AI might suggest an optimized production plan that levels workloads or shifts production to alternate plants to meet regional needs more efficiently. On the distribution side, AI can optimize warehouse operations and transportation. Companies like Amazon already use AI-driven robotics and algorithms to speed up order fulfillment in warehouses, but even outside of Amazon, many firms use AI for dynamic route optimization for deliveries or to predict delays and adjust logistics plans on the fly. The net effect is a more agile supply chain that can respond to changes quickly, with less manual fire-fighting.
Risks and Requirements
Of course, deploying AI in the supply chain isn’t plug-and-play. A key requirement is data – lots of it, and of good quality. AI models need access to data across sales, marketing, inventory, production, and even external sources. This means companies must break down data silos and possibly upgrade IT systems to integrate data sources. Data inaccessibility has historically been a major challenge in supply chains – if the AI can’t get the necessary data, its predictions will be limited or skewed. So, one of the first steps is often ensuring a clean, accessible data pipeline.
Despite these challenges, the trend is clear: companies that get supply chain AI right can achieve a more predictive and proactive operation. We’re moving from supply chains that react to yesterday’s numbers to supply networks that anticipate tomorrow’s needs. In the manufacturing sector, where margins can be thin and competition high, this can be a game-changer. Perhaps just as important as the technology is the process of updating skills and processes – operations managers and supply chain analysts need training to work effectively with AI tools, interpreting results and maintaining models. But as these capabilities mature, we are seeing manufacturing supply chains become far more efficient and resilient with the help of AI.
Process Optimization & Automation (Robotics Integration)
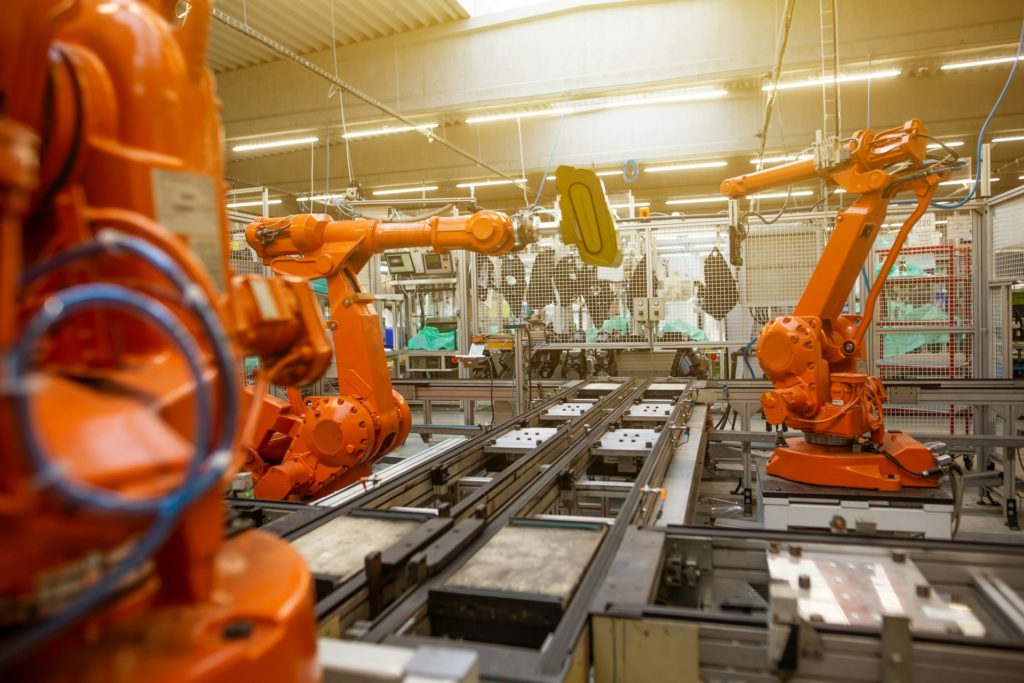
AI-driven automation is enabling robots and machines to adapt in real time, optimizing production processes without human intervention.
Identifying the Inefficiencies
Inside the factory, beyond quality inspection and planning, there lies a myriad of processes – assembly, machining, welding, packaging – where small inefficiencies can add up to big losses. Traditionally, manufacturing processes are optimized through lean methods and human engineering: engineers study the line, find bottlenecks, and reconfigure as needed. But even well-optimized lines face challenges like unplanned downtime, suboptimal scheduling, equipment sitting idle, or processes that aren’t tuned to peak efficiency. For example, a production line might run below capacity because it’s limited by the slowest station, or machines might not be calibrated to optimal settings, resulting in slower cycle times or more wear and tear. Changeovers are often lengthy and not well-optimized. Moreover, as demand patterns shift, a static production plan can lead to some lines overburdened while others are underutilized. Essentially, without advanced help, factories can leave a lot of potential productivity on the table.
How AI Supports Automation & Decision-Making
AI in process optimization takes many forms, but a common thread is using algorithms to make real-time decisions that tune and adapt the production process for optimal performance. One of the most visible aspects is robotics integration. Modern industrial robots are increasingly outfitted with AI capabilities – from computer vision that allows a robot to “see” and handle varied parts, to machine learning that lets robots refine their movements for speed and precision. For instance, AI algorithms can enable robotic arms to adjust their path dynamically if a part is slightly misaligned on a conveyor, rather than halting the line. This flexibility is a step beyond traditional robots that rigidly follow pre-programmed paths. In one case study, an AI system trained robots for intricate assembly tasks using simulations and achieved a doubling of production throughput, demonstrating how AI-trained robotics can dramatically speed up operations.
Beyond robotics, AI is optimizing processes through advanced analytics and control algorithms. A concept known as the “self-optimizing factory” is emerging, where sensors throughout the production line feed data into AI systems that continuously find the best settings. Think of an injection molding machine that produces plastic parts: AI can analyze temperature, pressure, and output quality data in real time and tweak the machine’s settings on the fly to keep quality high while minimizing cycle time. Similarly, in a complex assembly operation, AI might analyze the work in progress and decide to resequence tasks or deploy an extra robotic helper to a busy station if it detects a bottleneck forming.
Another area is predictive maintenance and scheduling – ensuring that equipment downtime is minimized and scheduled optimally. AI models can predict when a machine is likely to fail or need service and schedule maintenance during planned downtime, not when the machine is in the middle of a production run. This prevents those nasty unplanned breakdowns that can halt an entire line. By predicting failures in advance, AI helps maintain consistent process flow and can recommend the best times to service equipment to have the least impact on overall output.
AI is also being used for production scheduling optimization. In a factory with multiple product lines and products, figuring out the optimal production schedule is a complex optimization problem with many constraints. Traditional scheduling often uses heuristics or simplistic algorithms. AI can crunch these variables and come up with schedules that maximize throughput and minimize downtime or changeover penalties. The result is more orders fulfilled on time and better equipment utilization.
Tangible Gains in Output and Efficiency
The ultimate promise of AI-driven process optimization is higher productivity, lower costs, and greater flexibility on the factory floor. And we’re seeing this play out in practice. This comes from various sources – faster cycle times, less downtime, better yield, and finer-tuned processes. For example, Siemens applied AI in its own factories for process improvements and reported significant outcomes. In one Siemens facility, introducing AI for predictive maintenance and energy management contributed to a 20% reduction in energy consumption and drastically cut unplanned downtime, saving millions of euros annually. While energy usage is slightly tangential to core production, it shows that AI can optimize even the support processes in production to save costs.
On the direct production side, throughput gains are a major win. An automotive manufacturer integrating AI and robotics saw production efficiency improve and defect rates drop, which effectively means more sellable cars per day from the same line. AI helped their robots and quality systems work in concert – when quality issues were detected, the system fine-tuned robot operations to correct the issue, maintaining flow without manual intervention. In another instance, a manufacturer using an AI scheduling system managed to increase on-time delivery and keep their machines running closer to full capacity; essentially, AI found ways to produce the same orders in less time by optimizing sequence and resource allocation.
Flexibility is a benefit that’s a bit harder to quantify but incredibly important in today’s market. AI and advanced automation give factories the ability to adapt to changes more readily. If there’s a sudden priority order, an AI-optimized operation might be able to insert that order into the sequence with minimal disruption, something that would be very hard with static schedules. Or if a certain machine goes down, AI could quickly recompute an alternate plan to use other machines or routes, minimizing the impact. This kind of resilience and agility is increasingly seen as a competitive advantage – it’s not just about raw output, but the ability to dynamically optimize when conditions change.
The integration of AI also means better use of resources. Robots and machines can operate with less oversight, which can reduce labor costs or allow the workforce to focus on higher-level tasks. It’s not about replacing people so much as repurposing human talent – instead of manually moving materials or performing a routine task, workers can supervise a fleet of AI-guided robots or focus on tasks that require human creativity and problem-solving. Many factories are adopting a model where humans and AI-driven machines work in tandem: AI handles the repetitive optimization and real-time adjustments, while humans handle exceptions, improvements, and multi-faceted tasks.
Integration Challenges
Achieving these gains is not without challenges. One big hurdle is the integration of AI with legacy equipment and systems. Many factories have machines that are decades old which weren’t designed to stream data or be controlled by external algorithms. Retrofitting sensors and connecting these machines to an IIoT (Industrial Internet of Things) network is often a prerequisite. This can be technically challenging and expensive. Manufacturers must often decide whether to upgrade equipment to be “smart” or only optimize certain parts of the process that are already sensor-equipped. Interoperability is crucial – getting machines, robots, and AI controllers speaking the same language.
There’s also the aspect of workforce impact. Introducing robots and AI can cause concern among workers about job security and changes in job roles. Successful implementation usually involves investing in workforce training and clearly communicating the vision – for example, that robots will take over dangerous or repetitive tasks while humans move into supervisory or maintenance roles. In fact, companies like Siemens explicitly made workforce training part of their AI rollout, “developing programs to upskill employees in AI and robotics, empowering the workforce to effectively manage and interact with new technologies”. This kind of approach helps ease the transition and leverages AI as a tool to enhance human productivity, not just replace it.
Integrating AI Solutions in Manufacturing
Adopting AI solutions in manufacturing isn’t just about technology – it’s about laying the groundwork so that AI/ML can actually deliver value. Based on industry experiences, here are key considerations for manufacturing leaders and operations managers when integrating AI:
Data Infrastructure & Quality
Ensure you have the right data to feed AI algorithms. This may mean investing in IoT sensors on production equipment, consolidating disparate databases, and cleaning up data. AI is only as good as the data you give it. Breaking down data silos is crucial – if your maintenance department, production line, and supply chain teams all use separate systems that don’t talk, an AI can’t get the full picture. Many companies start by building a centralized data platform to gather inputs from across the operation. Additionally, establish data governance: define what data is collected, how it’s labeled, and how often it’s updated. Remember, if you train a model on flawed or biased data, the AI’s recommendations will also be flawed.
Pilot Projects with Clear ROI
It’s wise to start small with AI projects – pick a specific use case that has a clear problem and enough data. For example, you might start with an AI vision system on one troublesome quality station, or a pilot of AI forecasting for one product line. Define what success looks like and measure it. Pilot projects allow you to learn on a smaller scale, work out technical kinks, and build confidence within the organization. They also provide a proof-of-concept that can help secure buy-in for broader rollouts. When the pilot proves its ROI – say it saved a certain amount of cost or improved throughput – you can then scale that solution to other lines or plants. This iterative approach prevents massive sunk costs in case something doesn’t work as expected. We often see manufacturers run a pilot in one facility, then gradually expand to others once the approach is validated.
Workforce Upskilling and Buy-In
Integrating AI in the manufacturing sector requires a workforce that’s ready to work with new technology. Invest in training programs to upskill employees – from frontline operators learning to interpret AI system dashboards, to maintenance technicians learning how to handle predictive maintenance alerts, to analysts getting versed in data science basics. The culture should be one of humans plus AI, not humans versus AI. When Siemens rolled out AI, they coupled it with employee training and saw it as a way to “empower the workforce to manage and interact with new technologies”. Engage employees early, explain how AI tools will make their jobs safer or easier. Address fears by highlighting that there will always be a need for human judgment, oversight, and innovation – AI will handle repetitive tasks and number crunching, but humans are still in charge. Having champions or “AI ambassadors” on the factory floor – employees who are tech-savvy and enthusiastic – can help others get on board through peer learning.
Technology and Partner Selection
Decide whether you will build AI solutions in-house or partner with vendors. There are many AI solutions for manufacturing now available off the shelf – from computer vision platforms to predictive maintenance services to AI-driven planning software. Partnering can accelerate implementation, but ensure the solution fits your specific processes. Evaluate vendors not just on algorithms, but on how well they understand manufacturing operations. If building in-house, consider hiring experienced data scientists or partnering with local universities or consultants. Also, plan for IT integration – your AI tools must connect with existing factory systems. Open APIs and middleware might be needed. Don’t forget about scalability: choose solutions that can scale from one line to your entire enterprise if needed, and that can adapt as your business grows.
Ethics, Transparency, and Change Management
As AI takes on more decision-making, maintain transparency about how decisions are made. For critical areas, you may need AI systems that can explain their reasoning. This is important for trust and for debugging when things go wrong. Additionally, manage the change carefully – involve cross-functional teams from the start. The more collaboration across departments, the smoother the AI adoption. In fact, lack of cross-department coordination was cited as a top barrier to digital transformation for manufacturers. Overcome this by establishing clear governance for AI projects. Essentially, treat an AI initiative not just as a tech upgrade, but as a business process change that touches people, process, and technology.
By considering these factors – from having the right data infrastructure to nurturing the right skills and culture – manufacturers can greatly increase the odds of a successful AI integration. The companies that do this well tend to start small but think big: they create a roadmap where initial wins in AI lead to broader transformations in how they operate. And importantly, they keep the focus on business value: AI and ML in manufacturing are not implemented for bragging rights, but to solve real operational problems with measurable improvements.
Concluding Thoughts
AI in the manufacturing industry has moved from buzzword to practical toolset, delivering tangible improvements in quality, efficiency, and agility. The examples of AI in manufacturing we discussed – from a vision system catching nearly every defect to a machine learning model rebalancing a global supply chain – show that AI/ML isn’t a magic wand, but a powerful new toolkit for those who know how to use it. The manufacturing leaders, operations managers, and engineers who embrace these technologies are finding that they can run their factories with a level of insight and foresight that simply wasn’t possible before. We have AI systems acting as tireless inspectors, strategic planners, and expert process tuners, all working alongside humans to build better products at lower cost.
Looking forward, the question is not whether AI will be used in manufacturing – that’s already a given, as evidenced by the high adoption rates – but how well companies will harness its potential. The competitive frontier is shifting: just as lean manufacturing differentiated companies in the past, AI-enabled manufacturing will differentiate the factories of the future. We are likely to see more convergence of AI with other emerging technologies like IoT, cloud/edge computing, and even augmented reality.